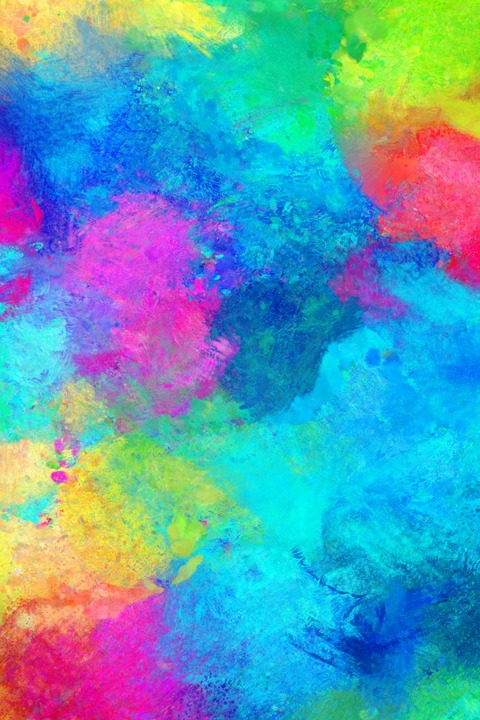
In the early days of deep learning, models were relatively simple, consisting of only a few layers of neurons. These models were limited in their ability to learn complex patterns and relationships in data, and often struggled with tasks that required a high degree of nuance and context. However, as researchers delved deeper into the field, they began to develop more advanced architectures that could handle the complexity of real-world data.
One of the key breakthroughs in the evolution of deep learning was the development of convolutional neural networks (CNNs) in the 1990s. CNNs are specifically designed to analyze visual data, such as images and videos, by taking into account the spatial relationships between pixels. This allowed for more accurate image recognition and classification, leading to advancements in fields such as computer vision and autonomous driving.
Another significant development in deep learning was the introduction of recurrent neural networks (RNNs), which are capable of processing sequential data, such as text and speech. RNNs have been instrumental in the development of natural language processing (NLP) models, enabling machines to understand and generate human language with a high degree of accuracy.
In recent years, the field of deep learning has seen the rise of transformer models, such as the famous BERT and GPT-3 models, which have pushed the boundaries of what is possible with artificial intelligence. These models are capable of processing vast amounts of text data and generating human-like responses, leading to advancements in chatbots, language translation, and content generation.
The evolution of deep learning has also been driven by advancements in hardware, such as the development of graphics processing units (GPUs) and specialized chips designed for neural network processing. These advancements have allowed researchers to train larger and more complex models, leading to significant improvements in performance and accuracy.
As deep learning continues to evolve, researchers are exploring new architectures, such as graph neural networks and attention mechanisms, that promise to further enhance the capabilities of AI systems. With each new breakthrough, the potential applications of deep learning expand, from healthcare and finance to entertainment and cybersecurity.
In conclusion, the evolution of deep learning has been characterized by a steady increase in complexity and capability, driven by advances in architecture, hardware, and research. As models continue to grow in sophistication, the possibilities for artificial intelligence are endless, promising a future where machines can truly understand and interpret the world around us.