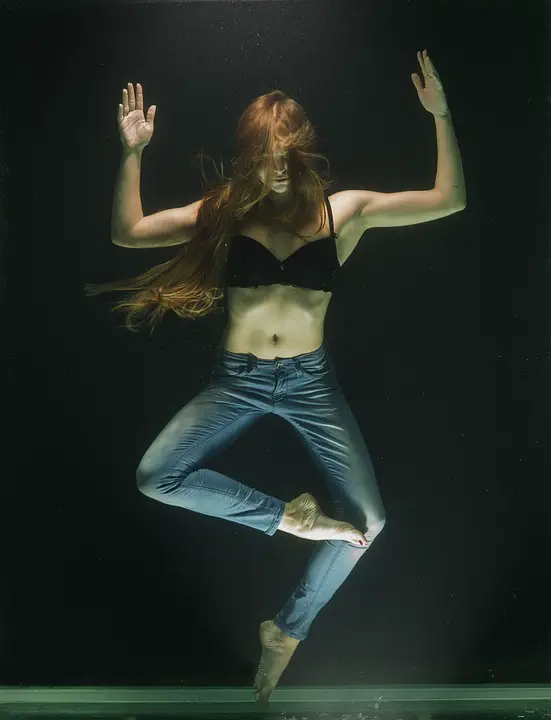
In recent years, the field of machine learning has seen a significant advancement with the rise of deep learning techniques. Deep learning, a subset of machine learning, is a powerful tool that has revolutionized the way we process and analyze data. From speech recognition to image classification, deep learning algorithms have proven to be highly effective in making sense of large and complex datasets.
The key to understanding the role of deep learning in machine learning lies in its ability to automatically learn and extract intricate patterns from raw data. Traditional machine learning algorithms require the manual extraction of features from data, which can be a time-consuming and labor-intensive process. Deep learning, on the other hand, uses neural networks to automatically learn the features and relationships within the data, making it a more efficient and accurate approach.
One of the most popular deep learning architectures is the convolutional neural network (CNN), which is commonly used in image recognition tasks. CNNs are designed to recognize patterns in visual data by applying filters to different parts of an image. This allows the network to learn features such as edges, shapes, and textures, leading to highly accurate image classification results.
Another widely used deep learning architecture is the recurrent neural network (RNN), which is often used in natural language processing tasks. RNNs are able to process sequential data by maintaining a memory of past inputs, making them well-suited for tasks such as language translation, sentiment analysis, and speech recognition.
The success of deep learning in machine learning can be attributed to the availability of large datasets and powerful computing resources. With the advent of big data and cloud computing, researchers and practitioners have access to vast amounts of data and computing power, enabling them to train complex deep learning models on massive datasets.
Despite its impressive capabilities, deep learning does have its limitations. One of the main challenges is the need for large amounts of labeled data to train the models effectively. This can be costly and time-consuming, especially in domains where labeled data is scarce or difficult to obtain. Additionally, deep learning models can be computationally expensive to train and require specialized hardware such as GPUs to achieve optimal performance.
In conclusion, deep learning plays a crucial role in machine learning by enabling us to make sense of complex and unstructured data. Its ability to automatically learn features from raw data has led to breakthroughs in various fields such as computer vision, natural language processing, and healthcare. As we continue to harness the power of deep learning, we can expect to see even more advancements in the way we extract insights and make decisions from data.