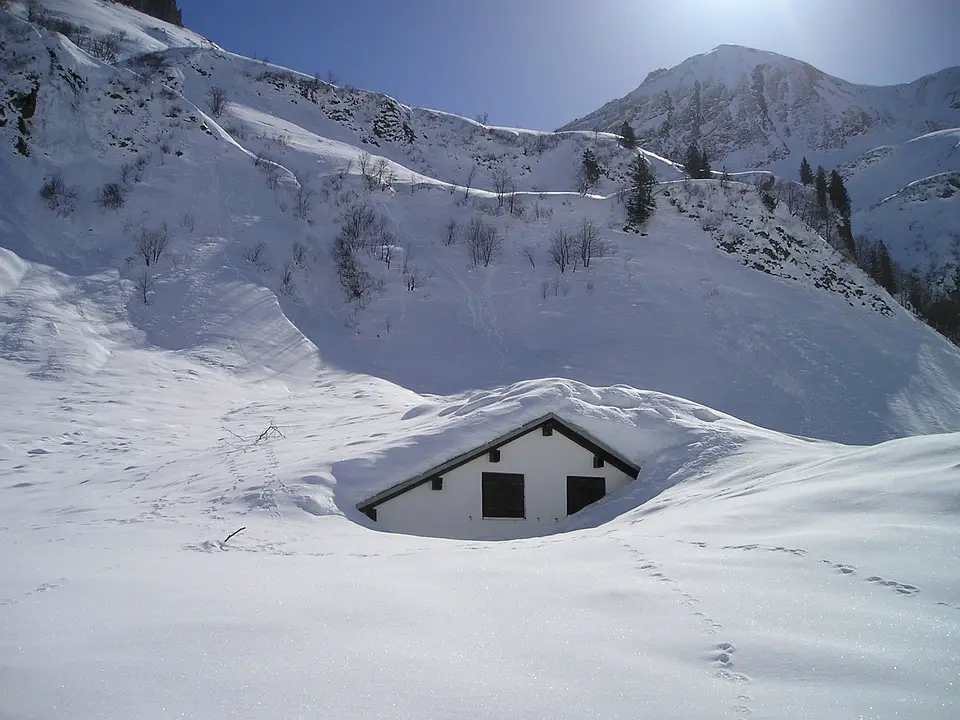
But what goes on behind the scenes of these deep learning models? How do they work and what makes them so effective? In this article, we will explore the technology behind deep learning models and shed some light on their inner workings.
At the core of deep learning models is a neural network, which is a mathematical model inspired by the structure of the human brain. A neural network consists of layers of interconnected nodes, or artificial neurons, that process and analyze data. Each neuron takes in input data, applies a mathematical operation to it, and passes the result to the next layer of neurons. By adjusting the weights of the connections between neurons, the neural network can learn to recognize patterns and make predictions.
Training a deep learning model involves feeding it a large amount of labeled data and adjusting the weights of the neural network through a process called backpropagation. During training, the model compares its predictions to the actual labels and calculates the error, or the difference between the predicted and actual values. The model then updates the weights of the connections between neurons to minimize this error, gradually improving its accuracy over time.
One of the key advantages of deep learning models is their ability to automatically extract features from the data, eliminating the need for manual feature engineering. Traditional machine learning algorithms require domain experts to identify relevant features in the data, which can be time-consuming and error-prone. Deep learning models, on the other hand, can learn to extract features directly from the raw data, making them more versatile and adaptable to different tasks.
Another important aspect of deep learning models is their ability to handle unstructured data, such as images, text, and audio. Convolutional neural networks (CNNs) are commonly used for image recognition tasks, while recurrent neural networks (RNNs) are used for sequential data such as text and speech. These specialized architectures enable deep learning models to process and analyze complex data types, opening up new possibilities for applications in computer vision, natural language processing, and speech recognition.
Despite their impressive capabilities, deep learning models also come with challenges and limitations. They require large amounts of labeled data to train effectively, which can be expensive and time-consuming to collect. Additionally, deep learning models are often considered black boxes, meaning that it can be difficult to interpret how they arrive at their predictions. This lack of transparency can be a barrier to adoption in industries where explainability is critical, such as healthcare and finance.
In conclusion, deep learning models have revolutionized the field of artificial intelligence and machine learning, enabling computers to learn complex patterns and make predictions with unprecedented accuracy. By leveraging neural networks and specialized architectures, deep learning models can process and analyze unstructured data, opening up new possibilities for applications in various fields. However, challenges such as the need for large amounts of labeled data and the lack of interpretability remain obstacles to widespread adoption. As researchers continue to push the boundaries of deep learning technology, we can expect to see even more impressive advancements in the years to come.