Unlock AI’s Full Potential: How to Overcome Enterprises’ Biggest Data and Infrastructure Challenges
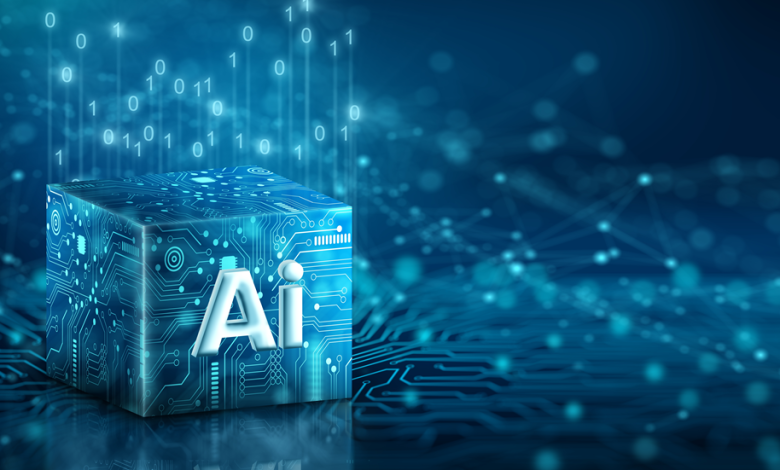
The exponential growth of data has transformed how enterprise organizations understand their customers, driving the need for advanced systems to manage massive data sets and deliver hyper-personalized experiences. While modern architectures, fueled by Moore’s Law, have replaced legacy systems, key challenges persist in scaling, reliability, and performance—further complicated by an increasingly privacy-focused, multi-cloud environment.
As AI models become more sophisticated and accessible, many companies are entering an AI “arms race.” To ensure success, companies must fortify their enterprise systems with more robust and higher-quality data.
Mobilize More Engineers, Data Scientists, and Analysts
AI, and more specifically, machine learning, isn’t new. For over a decade, these techniques have been growing in adoption to enhance the way enterprises interact with customers. Whether in marketing and customer service or in how product and engineering teams build solutions, ML and AI have been used to meet customers’ growing demands for convenience and performance.
So why all the hype now? AI has become democratized. The onset of tools, like ChatGPT, has greatly diminished the barrier to adoption, and faster and more affordable processing power allows new AI tools and techniques at a breakneck pace. Simpler interfaces, and AI’s ability to understand human language with techniques including large language models (LLMs), mean more people can use the technology to unlock innovation and new use cases. There are also many new application programming interfaces (APIs) and tools that make it possible to leverage AI models without having to understand the nitty-gritty of how they work.
Now that AI can “speak our language” in place of advanced mathematics, more engineers, data scientists, and analysts can leverage AI to drive transformation.
Fuel AI With a Data Foundation Built for Modern Demands
While AI has become more user-friendly, it can only reach its full potential with a foundation of high-quality, proprietary data built to scale with the modern enterprise. Technologists must solve challenges with scalability, reliability, and speed if they hope to feed transformative AI algorithms. Otherwise, the classic “garbage in, garbage out” principle will apply.
1. Scale with Modern Architectures: Introducing new workflows, or improving existing ones, is complex and puts large constraints on enterprise systems. This requires a complete overhaul of architectures using cutting-edge technologies such as more scalable distributed relational databases, or faster streaming frameworks.
For example, a company may wish to save valuable human time by building an AI-based classification system to detect sensitive data, which is critical in today’s privacy-centric world. To enable new AI use cases, a flexible architecture must be in place to accommodate the specific needs and data quality these tools require.
2. Unlock Multi-Cloud Through Data Federation: Enterprises avoid vendor lock-in to ensure flexibility and future-proofing in their tech stacks. They also want to maximize the value of their data and obtain more value from their partners’ data, yet often use a different cloud or data warehouse.
AI does best when multiple data sets are brought together to unearth more rich and diverse insights. Use a secure, federated approach to let AI access data across multiple clouds, which enables seamless data collaboration tailored to diverse business needs within and between enterprises.
3. Enhance Reliability with Measurement: “Always on” enterprise systems demand higher reliability and quality. AI demands massive amounts of data, which also necessitates seamless reliability — and scalability — of the pipelines, observability, real-time problem-solving, and automation.
If this reliability is absent, AI will require frequent human intervention for basic data operations, slowing innovation and dampening results. Metrics-driven approaches and comprehensive system observability make this achievable by ensuring real-time problem-solving and robust automation checks.
4. Optimize for Speed: Enterprises need rapid data processing as data volumes surge. By modernizing architectural approaches and leveraging new technologies like high-scale, distributed relational databases, businesses can achieve faster data turnaround times, balancing cost and efficiency.
AI has historically been expensive and compute-heavy. If costs are a concern, enterprises should be selective about prioritizing the use cases that unlock quantifiable business value, and understand which data is required for those algorithms, as processing systems catch up.
Drive Innovation Through Creativity and Experimentation
The most important element of AI success is creativity. Maintaining an experimental mindset will allow companies to explore new use cases and fuel innovation.
First, don’t be afraid to try lots of things. Run experiments to determine what’s possible and what’s of value. For example, ask AI to convert a question to a structured query language (SQL) query. Run it against a dataset to generate new insights, or gain a deeper understanding of marketing data to finetune campaign performance.
Next, identify the 10 most promising experiments with the most business value and low barriers to build. They may require alpha or beta tests, but customer feedback can improve them. Take an AI customer assistant, for instance. It can be used to help clients configure a workflow to get the results they want, such as a better understanding of what’s happening in a product, or how new capabilities can be utilized.
Finally, formally adopt the use cases where friction is low and repeatability is high. These are the experiments that have been proven, incorporating customer feedback, and can move quickly.
Regardless of your company’s goals, build your AI strategy with an experimental mindset. Know you don’t know everything, and that’s okay. Having a flexible, open data architecture that can accommodate rapid experimentation and fast deployment is how to lay the modern foundation for future success — the “next ChatGPT” could arrive unexpectedly and explosively at any moment. The speed of innovation is high. Take advantage of it.
About the Author
Mohsin Hussain is the Chief Technology Officer at LiveRamp (NYSE: RAMP), leading the global engineering team. In his role, Mohsin is responsible for ensuring LiveRamp’s world-class products and technology platforms continue to scale and innovate to meet the ever-growing needs of the company’s 1000+ customers across a broad range of sectors including brands, agencies, technology vendors, data providers, and publishers. With more than 25 years of experience in engineering, leadership, and innovation, he brings an extensive technical background in software and distributed systems, data science and machine learning, analytics and the cloud. His career has spanned leadership roles across several high-growth start-ups and public companies including AOL/Netscape, Seibel, SunPower, and Criteo. Mohsin has expertise building large-scale high-availability systems, cultivating empowerment-based engineering culture, and integrating complex technology M&A.
Sign up for the free insideAI News newsletter.
Join us on Twitter: https://twitter.com/InsideBigData1
Join us on LinkedIn: https://www.linkedin.com/company/insideainews/
Join us on Facebook: https://www.facebook.com/insideAINEWSNOW