This AI Paper by NVIDIA Introduces NVLM 1.0: A Family of Multimodal Large Language Models with Improved Text and Image Processing Capabilities

Multimodal large language models (MLLMs) focus on creating artificial intelligence (AI) systems that can interpret textual and visual data seamlessly. These models aim to bridge the gap between natural language understanding and visual comprehension, allowing machines to cohesively process various forms of input, from text documents to images. Understanding and reasoning across multiple modalities is becoming crucial, especially as AI moves towards more sophisticated applications in areas like image recognition, natural language processing, and computer vision. By improving how AI integrates and processes diverse data sources, MLLMs are set to revolutionize tasks such as image captioning, document understanding, and interactive AI systems.
A significant challenge in developing MLLMs is ensuring they perform equally well on text-based and vision-language tasks. Often, improvements in one area can lead to a decline in the other. For instance, enhancing a model’s visual comprehension might negatively affect its language capabilities, which is problematic for applications requiring both, such as optical character recognition (OCR) or complex multimodal reasoning. The key issue is balancing processing visual data, like high-resolution images, and maintaining robust text reasoning. As AI applications become more advanced, this trade-off becomes a critical bottleneck in the progress of multimodal AI models.
Existing approaches to MLLMs, including models such as GPT-4V and InternVL, have tried to address this problem using various architectural techniques. These models freeze the language model during training or employ cross-attention mechanisms to process image and text tokens simultaneously. However, these methods are not without flaws. Freezing the language model during multimodal training often results in poorer performance on vision-language tasks. In contrast, open-access models like LLaVA-OneVision and InternVL have shown marked degradation in text-only performance after multimodal training. This reflects a persistent issue in the field, where advancements in one modality come at the cost of another.
Researchers from NVIDIA have introduced the NVLM 1.0 models, representing a significant leap forward in multimodal language modeling. The NVLM 1.0 family consists of three main architectures: NVLM-D, NVLM-X, and NVLM-H. Each of these models addresses the shortcomings of prior approaches by integrating advanced multimodal reasoning capabilities with efficient text processing. A noteworthy feature of NVLM 1.0 is the inclusion of high-quality text-only supervised fine-tuning (SFT) data during training, which allows these models to maintain and even improve their text-only performance while excelling in vision-language tasks. The research team highlighted that their approach is designed to surpass existing proprietary models like GPT-4V and open-access alternatives such as InternVL.
The NVLM 1.0 models employ a hybrid architecture to balance text and image processing. NVLM-D, the decoder-only model, handles both modalities in a unified manner, making it particularly adept at multimodal reasoning tasks. NVLM-X, on the other hand, is built using cross-attention mechanisms, which enhance computational efficiency when processing high-resolution images. The hybrid model, NVLM-H, combines the strengths of both approaches, allowing for more detailed image understanding while preserving the efficiency needed for text reasoning. These models incorporate dynamic tiling for high-resolution photos, significantly improving performance on OCR-related tasks without sacrificing reasoning capabilities. Integrating a 1-D tile tagging system allows for accurate image token processing, which boosts performance in tasks like document understanding and scene text reading.
Regarding performance, the NVLM 1.0 models have achieved impressive results across multiple benchmarks. For instance, on text-only tasks like MATH and GSM8K, the NVLM-D1.0 72B model saw a 4.3-point improvement over its text-only backbone, thanks to integrating high-quality text datasets during training. The models also demonstrated strong vision-language performance, with accuracy scores of 93.6% on the VQAv2 dataset and 87.4% on AI2D for visual question answering and reasoning tasks. In OCR-related tasks, the NVLM models significantly outperformed existing systems, scoring 87.4% on DocVQA and 81.7% on ChartQA, highlighting their ability to handle complex visual information. These results were achieved by the NVLM-X and NVLM-H models, which demonstrated superior handling of high-resolution images and multimodal data.
One of the key findings of the research is that the NVLM models not only excel in vision-language tasks but also maintain or improve their text-only performance, something that other multimodal models struggle to achieve. For example, in text-based reasoning tasks like MMLU, NVLM models maintained high accuracy levels, even surpassing their text-only counterparts in some cases. This is particularly important for applications that require robust text comprehension alongside visual data processing, such as document analysis and image-text reasoning. The NVLM-H model, in particular, strikes a balance between image processing efficiency and multimodal reasoning accuracy, making it one of the most promising models in this field.
In conclusion, the NVLM 1.0 models developed by researchers at NVIDIA represent a significant breakthrough in multimodal large language models. By integrating high-quality text datasets into multimodal training and employing innovative architectural designs like dynamic tiling and tile-tagging for high-resolution images, these models address the critical challenge of balancing text and image processing without sacrificing performance. The NVLM family of models not only outperforms leading proprietary systems in vision-language tasks but also maintains superior text-only reasoning capabilities, marking a new frontier in the development of multimodal AI systems.
Check out the Paper. All credit for this research goes to the researchers of this project. Also, don’t forget to follow us on Twitter and join our Telegram Channel and LinkedIn Group. If you like our work, you will love our newsletter..
Don’t Forget to join our 50k+ ML SubReddit
⏩ ⏩ FREE AI WEBINAR: ‘SAM 2 for Video: How to Fine-tune On Your Data’ (Wed, Sep 25, 4:00 AM – 4:45 AM EST)
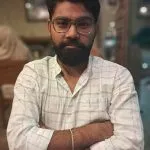
Nikhil is an intern consultant at Marktechpost. He is pursuing an integrated dual degree in Materials at the Indian Institute of Technology, Kharagpur. Nikhil is an AI/ML enthusiast who is always researching applications in fields like biomaterials and biomedical science. With a strong background in Material Science, he is exploring new advancements and creating opportunities to contribute.