Seven Factors Driving AI Adoption
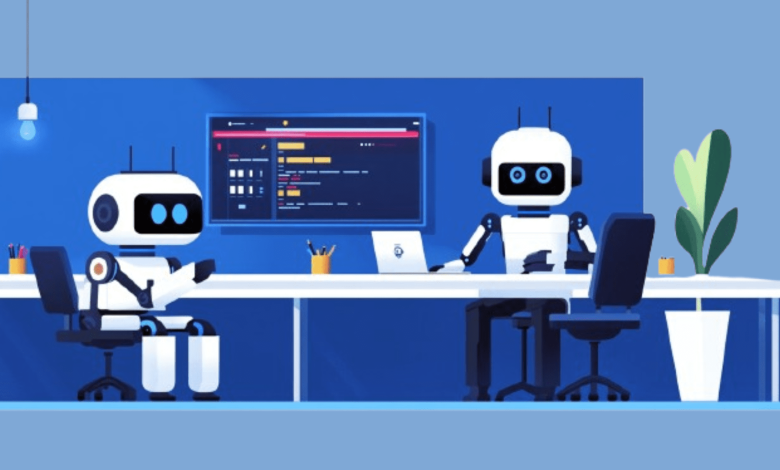
Image by Editor | Midjourney
There were days when algorithms crafting art and creating music were considered a work of science-fiction but not anymore. AI is no longer a future, it is a reality.
It is considered a transformative, iPhone moment — one which has the potential to reshape industries and transform business models. It has created excitement among companies to explore the state-of-the-art possible by leveraging the new phase of AI, i.e. generative AI.
As the industry is en route to reaping the benefits of this technological revolution, in this article, I’ll share seven factors driving AI adoption that cover the complexities driving AI adoption.
1. Start with a Clear Vision
One of the key factors to ensure AI initiatives find adoption is to start with building a clear, strategic vision. AI-ready organizations do not think of AI as a plug-and-play solution; rather, they understand that its implementation requires a deep knowledge of identifying the right opportunities where it can add value. Take, for example, successful organizations like Amazon and Netflix — their success in AI is mostly rooted in aligning AI initiatives with their core business objectives, be it to improve customer experience, optimize operations, or create new products.
This strategic approach to AI must ensure that the AI projects are connected to measurable business outcomes. This tangible outcome-based approach helps prioritize the high-impact use of AI.
2. Investments in Data Infrastructure
Data is the engine that drives quality AI outcomes. Let me refine this statement further: good quality data drives quality AI outcomes.
The data can come from various sources and is present in different shapes and forms. So, to gauge meaningful business insights, organizations require access to clean and organized data. This raises the need to timely invest in a scalable, future-proof data infrastructure that helps integrate data, providing a holistic view of business operations.
Such investments ensure effective data management practices and cloud-based solutions that provide the necessary scale as business requirements grow and evolve.
3. Develop Cross-Functional Teams
AI projects require extensive cross-functional collaboration involving data scientists, engineers, domain experts, and business leaders. Building cross-functional teams provides the much-needed fusion of technical expertise and business needs. In cases where business problems are not well understood, even the most effective technical solutions struggle to find adoption as they fail to cater to specific business needs.
4. Culture of Experimentation
AI projects are scientific which implies they are experimental in nature. They require several iterations, undergo rigorous testing, and continuous learning.
The companies that are best positioned to build scalable AI systems typically show the following characteristics:
- Foster a culture of experimentation
- Equate failure as a learning
- Encourage pivoting as needed, rather than giving into sunk-cost fallacy
- Promote the inquisitiveness to innovate
- Test existing approaches to solving problems
- Challenge the status quo, and
- Find which models work best
Such a flexible culture helps organizations build an innovation muscle.
5. Ethical and Responsible AI
With the increasing use of AI driving our decisions, the industry has come to realize the importance of building AI responsibly, which should ensure that the systems do not mirror or amplify biases and inequalities. There has been an increased focus on embedding ethical AI by design.
Several governance frameworks exist that provide overview for bias detection, and promote transparent and accountable AI systems.
Building ethical AI isn’t only about reputational risk; it’s about upholding the trust with technology, particularly in high-risk, high-impact sectors like healthcare, finance, and hiring.
6. Upskill Your Workforce
Taking your teams along is key to successful AI adoption. Clear, transparent, and timely communications help prepare your workforce for the AI transformation. Address their concerns whether it is about the fear of losing jobs or resistance to change that comes with such transformation.
Share how AI, similar to any other tool, augments decision-making rather than replaces human judgment.
Your team is your biggest leverage in successful AI implementation. Therefore, it is important to make reskilling and upskilling efforts to train them and onboard them in this transformative journey,
Forward-thinking organizations actively invest in AI training and literacy across the board building the lens to identify AI opportunities and also understand its limitations.
7. Continuous Journey
AI projects are not a one-time effort of building and deploying the model. Instead, they involve a continuous effort to maintain the model’s performance. Therefore, it is recommended to start small and prove business before planning to scale them incrementally. This phased approach allows taking your stakeholders along with, providing timely feedback and resolving any challenges when scaling.
Now, this is where it gets interesting. Let me share another dimension of the continuous journey AI technologies are constantly evolving, and organizations should keep themselves agile to adapt to these changes quickly. And, this agility is not just limited to organizations, even AI teams must stay updated with the latest AI advancements to build effective and efficient models.
In the end, I’d like to highlight that there is no rulebook that guarantees successful AI adoption. Every organization is uniquely placed to leverage technology and navigate its challenges as well as opportunities. Consider the factors shared in this article as your starting point to build a tailored approach that works best for your organization.
I hope your organization can maximize the impact of AI initiatives by following these best practices starting with the right use of AI, building data foundations to fostering a responsible AI culture.
Vidhi Chugh is an AI strategist and a digital transformation leader working at the intersection of product, sciences, and engineering to build scalable machine learning systems. She is an award-winning innovation leader, an author, and an international speaker. She is on a mission to democratize machine learning and break the jargon for everyone to be a part of this transformation.