Investing in AI to build next-generation infrastructure
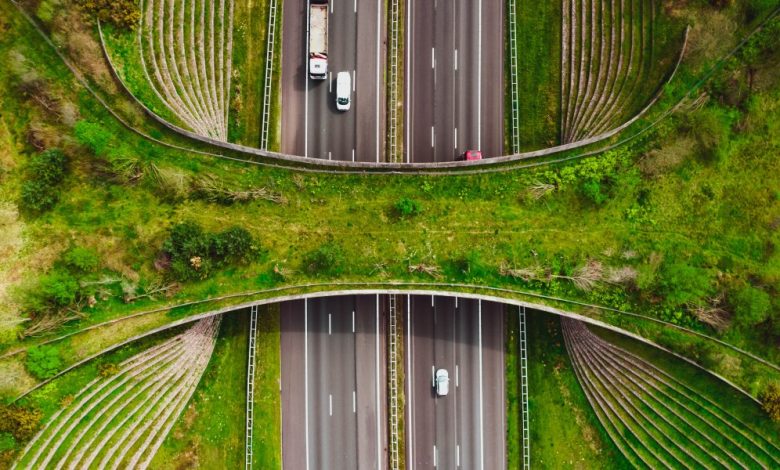
This infrastructure gap – the difference between funding and construction – is vast. And while governments and companies everywhere are feeling the strain of constructing an energy efficient and sustainable built environment, it’s proving more than humans can do alone. To redress this imbalance, many organizations are turning to various forms of AI, including large language models (LLMs) and machine learning (ML). Collectively, they are not yet able to fix all current infrastructure problems but they are already helping to reduce costs, risks, and increase efficiency.
Overcoming resource constraints
A shortage of skilled engineering and construction labor is a major problem. In the US, it is estimated that there will be a 33% shortfall in the supply of new talent by 2031, with unfilled positions in software, industrial, civil and electrical engineering. Germany reported a shortage of 320,000 science, technology, engineering, and mathematics (STEM) specialists in 2022 and another engineering powerhouse, Japan, has forecast a deficit of more than 700,000 engineers by 2030. Considering the duration of most engineering projects (repairing a broken gas pipeline for example, can take decades), the demand for qualified engineers will only continue to outstrip supply unless something is done.
Immigration and visa restrictions for international engineering students, and a lack of retention in formative STEM jobs, exert additional constraints. Plus, there is the issue of task duplication which is something AI can do with ease.
Julien Moutte, CTO of Bentley Systems explains, “There’s a massive amount of work that engineers have to do that is tedious and repetitive. Between 30% to 50% of their time is spent just compressing 3D models into 2D PDF formats. If that work can be done by AI-powered tools, they can recover half their working time which could then be invested in performing higher value tasks.”
With guidance, AI can automate the same drawings hundreds of times. Training engineers to ask the right questions and use AI optimally will ease the burden and stress of repetition.
However, this is not without challenges. Users of ChatGPT, or other LLMs, know the pitfalls of AI hallucinations, where the model can logically predict a sequence of words but without contextual understanding of what the words mean. This can lead to nonsensical outputs, but in engineering, hallucinations can sometimes be altogether more risky. “If a recommendation was made by AI, it needs to be validated,” says Moutte. “Is that recommendation safe? Does it respect the laws of physics? And it’s a waste of time for the engineers to have to review all these things.”
But this can be offset by having existing company tools and products running simulations and validating the designs using established engineering rules and design codes which again relieves the burden of having the engineers having to do the validating themselves.
Improving resource efficiency
An estimated 30% of building materials, such as steel and concrete, are wasted on a typical construction site in the United States and United Kingdom, with the majority ending up in landfills, although countries such as Germany and The Netherlands have recently implemented recycling measures. This, and the rising cost of raw materials, is putting pressure on companies to think of solutions to improve construction efficiency and sustainability.