A data bottleneck is holding AI science back, says new Nobel winner
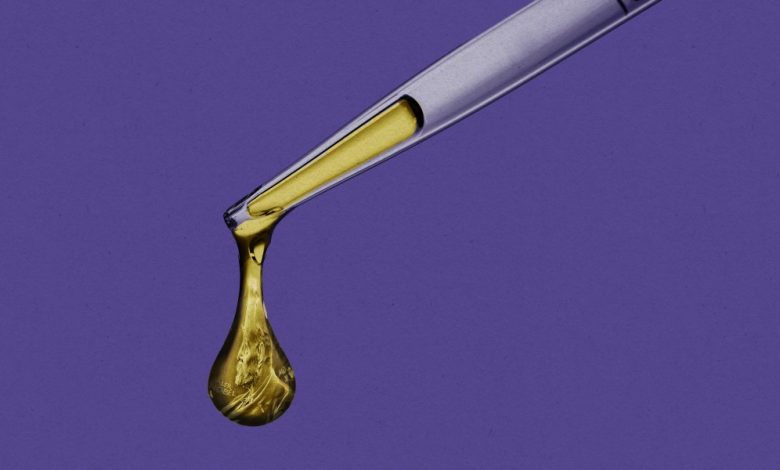
AI has been a gamechanger for biochemists like Baker. Seeing what DeepMind was able to do with AlphaFold made it clear that deep learning was going to be a powerful tool for their work.
“There’s just all these problems that were really hard before that we are now having much more success with thanks to generative AI methods. We can do much more complicated things,” Baker says.
Baker is already busy at work. He says his team is focusing on designing enzymes, which carry out all the chemical reactions that living things rely upon to exist. His team is also working on medicines that only act at the right time and place in the body.
But Baker is hesitant in calling this a watershed moment for AI in science.
In AI there’s a saying: Garbage in, garbage out. If the data that is fed into AI models is not good, the outcomes won’t be dazzling either.
The power of the Chemistry Nobel Prize-winning AI tools lies in the Protein Data Bank (PDB), a rare treasure trove of high-quality, curated and standardized data. This is exactly the kind of data that AI needs to do anything useful. But the current trend in AI development is training ever-larger models on the entire content of the internet, which is increasingly full of AI-generated slop. This slop in turn gets sucked into datasets and pollutes the outcomes, leading to bias and errors. That’s just not good enough for rigorous scientific discovery.
“If there were many databases as good as the PDB, I would say, yes, this [prize] probably is just the first of many, but it is kind of a unique database in biology,” Baker says. “It’s not just the methods, it’s the data. And there aren’t so many places where we have that kind of data.”
Deeper Learning
Adobe wants to make it easier for artists to blacklist their work from AI scraping